Events
ECE Imaging Seminar: Physics-Inspired Deep Learning for Inverse Problems in MRI
Nalini Singh, PhD Candidate, MIT
-The University of Texas at Austin EER 3.640
United States
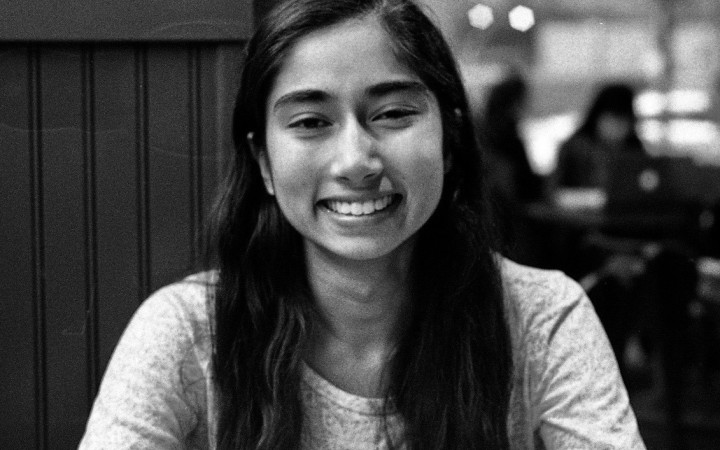
Abstract:
We demonstrate the power of combining the forward image acquisition model with deep learning solutions for inverse MRI problems, from individual network layers to the network architecture design and inference procedure.
First, we propose neural network layers that combine image space representations with representations in Fourier space, where MRI data is acquired. These layers can be used as drop-in replacements for standard image space convolutions in a variety of network architectures and yield higher quality reconstructions across a wide range of MR imaging tasks. Second, we demonstrate a deep learning framework for MRI motion correction. Our approach reduces the joint image-motion parameter search in most motion correction strategies to an inference-time search over motion parameters alone, greatly simplifying the complexity of the optimization problem to be solved for a novel image. The motion parameter search is guided by the physics of the forward imaging model and achieves high reconstruction fidelity while retaining the benefits of explicit model-based optimization – in particular, the ability to reject examples where the network produces poor reconstructions. This procedure systematically yields a reconstructed image and estimated motion parameters that are consistent with the acquired data.
Our experiments demonstrate the advantages of this combined approach over purely learning or model-based reconstruction techniques.
Bio: Nalini Singh is a final-year PhD student at the Harvard-MIT Division of Health Sciences and Technology, advised by Professor Polina Golland. Her research focuses on imaging inverse problems. Previously, she earned a Bachelor's degree in Electrical Engineering and Computer Science from MIT and was a student researcher at Google. She is the recipient of an NSF Graduate Research Fellowship, Google PhD Fellowship, and an outstanding reviewer award at CVPR and ICLR.