Events
GenAI Imaging Seminar: Learning‐based Artifacts Removing Priors for Computational Imaging
Jiaming Liu, PhD Candidate, Washington University in St. Louis
-The University of Texas at Austin
EER 3.640/3.642
United States
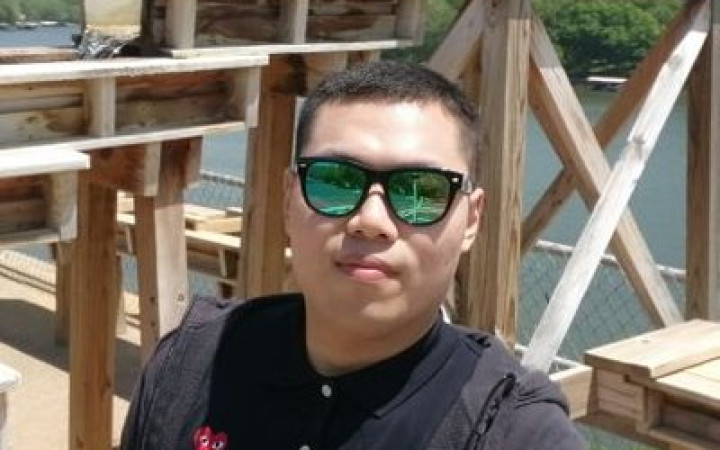
Abstract: Fast and robust recovery of images from a deluge of incomplete and noisy data is now within reach for many important biomedical applications. Success in such recovery tasks requires accurate and scalable algorithms that can leverage the knowledge of physics as well as strong statistical priors. For example, in compressed sensing magnetic resonance imaging (CS‐MRI), the physics model is used to ensure reliability, while the sparse nature of medical images is used as a prior to accelerate data acquisition. Recently, deep learning methods have shown state‐of‐the‐art results in a number of biomedical imaging tasks. Although powerful, the corresponding methods are often heuristic and fragile to outliers. In fact, there is still a lack of a robust and interpretable deep‐learning‐based framework that comes with provable theoretical guarantees. My research aims to bridge this gap by building a unified framework that is rapid, scalable to large‐scale data, and comes with provable guarantees. To achieve this goal, I utilize "artifacts removing" as a mechanism to incorporate prior knowledge into the recovery problem while keeping the prior explicitly decoupled from the data acquisition model. Hence, by using a "learned artifacts remover," my methodology can fully leverage the rich prior information in the training data while having the physics model accommodate outliers. We demonstrate the performance of our algorithms by validating their performance on various imaging applications and providing rigorous theoretical analysis. This presentation evaluates and outlines my past work to explore three specific topics: (a) image reconstruction using artifacts removing priors for 4D free‐breathing MRI; (b) efficient deep learning for data‐intensive biomedical imaging with theoretical guarantees; (c) deep probabilistic priors for high‐ dimensional inverse imaging, all of which are centered on developing new algorithms and mathematical tools for advanced computational imaging applications.
Speaker Bio: Jiaming Liu received the B.Sc. degree in electronic and information engineering from the University of Electronic Science and Technology of China, Chengdu, China, and the M.S. degree in electrical and engineering from Washington University in St. Louis, St. Louis, MO, USA, where he is currently working toward the Ph.D. degree with the Computational Imaging Group. His research interests include computational biomedical imaging, machine learning, and optimization.
The seminar is hosted by the UT Computational Sensing and Imaging Lab in collaboration with the Center for Generative AI.