Events
Use-Inspired Research Seminar
IFML Seminar: How to Measure the Depth in a Fully Connected Network?
Boris Hanin, Princeton
-The University of Texas at Austin
Gates Dell Complex (GDC 6.302)
United States
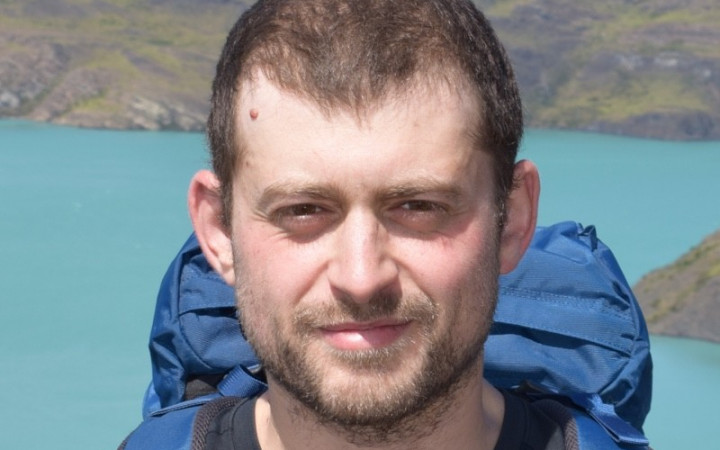
Abstract: A neural network with L = 0 hidden layer is simply a linear model. In contrast, networks with L large are typically considered highly non-linear. However, at any fixed value of L networks at infinite width become linear models in two senses:
- Using standard initialization schemes the outputs are independent Gaussians precisely as they would be in a linear model at initialization
- No feature learning occurs: the supposedly non-linear network can be replaced by its linearization at the start of training
This suggests that L alone is not a satisfactory measure of network depth. For fully connected networks with hidden layer widths proportional to a large parameter n, I will argue that the correct measure of network depth is the depth-to-width ratio L / n. A variety of Theorems will show that large values of n make neural networks more like Gaussian processes with independent components, which are well behaved but incapable of feature learning (at least with standard initialization schemes). Large values of L, in contrast, amplify higher cumulants and inter-neuron correlations as well as changes in the NTK, both of which scale with the network aspect ratio L/n. Based on joint work with Dan Roberts, Sho Yaida, Mihai Nica, and David Rolnick.
Speaker Bio: Boris Hanin has been an Assistant Professor at Princeton ORFE since Fall 2020, and his research is on machine learning, probability, and mathematical physics. Prior to Princeton, he was an Assistant Professor in Mathematics at Texas A&M. He has also held visiting positions at Google, Facebook AI, and the Simons Institute.
Event RegistrationSpeaker Bio: Boris Hanin has been an Assistant Professor at Princeton ORFE since Fall 2020, and his research is on machine learning, probability, and mathematical physics. Prior to Princeton, he was an Assistant Professor in Mathematics at Texas A&M. He has also held visiting positions at Google, Facebook AI, and the Simons Institute.