Events
ML+ X Seminar
Multi-Modal Deep Learning of Electrocardiograms for Precision Cardiovascular Health
Benjamin Glicksberg, Assistant Professor, Icahn School of Medicine at Mount Sinai
-The University of Texas at Austin
Gates Dell Complex (GDC 6.302)
2317 SPEEDWAY
Austin , TX 78712
United States
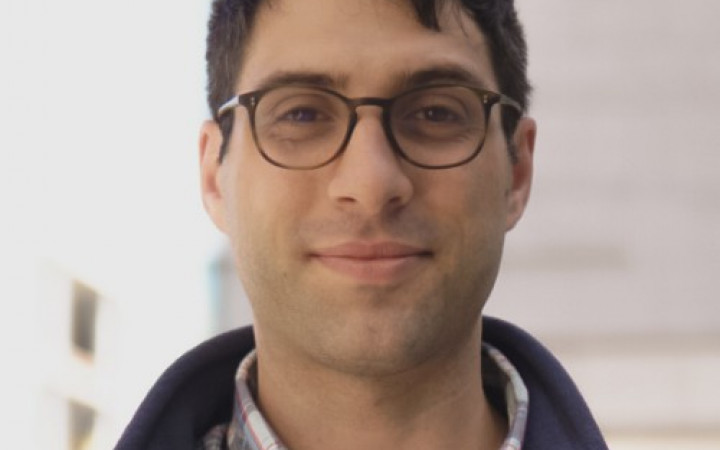
Watch the replay here.
Abstract: Artificial intelligence continues to permeate healthcare but has been met with mixed success. While the digitization of the patient has led to important discoveries, these data have their own limitations and biases which restrict the utility of the machine learning models that are built on them. The utilization of multiple modalities of digital patient data in modeling can alleviate some of these issues but their discrepant structure make effective fusion challenging. This talk will highlight opportunities and challenges of creating multi-modal machine learning models in multiple clinical domains. To showcase the power of these approaches, projects from two recent publications will be highlighted that target clinical questions from different angles. The first project highlights how deep neural networks using a massive corpus of Electronic Health Records (EHR) and raw electrocardiogram (ECG) waveform data can be used to more quickly detect clinically-relevant heart failure conditions that often require extensive follow-up. In the second, deep fusion models of EHR and ECG data are used to increase screening specificity for pulmonary embolism for moderate-to-high suspicion individuals. This work hints at the possibility of reducing testing burden for a disease that is hard to accurately screen. Finally, recent work leveraging federated learning approaches will be highlighted as ways to overcome potential health system-specific biases and create more robust, generalizable models for more personalized medicine.
Speaker Bio: Benjamin Glicksberg, Ph.D. is an assistant professor at the Icahn School of Medicine at Mount Sinai in the departments of AI in Human Health; Medicine; and Genetics and Genomic Sciences, and a member of the Hasso Plattner Institute for Digital Health. He is also an adjunct faculty member of digital engineering at the Hasso Plattner Institute in Potsdam, Germany. His research uses machine learning to couple multi-modal patient health data to forward personalized medicine. He completed his Ph.D. in Neuroscience at the Icahn School of Medicine at Mount Sinai in 2017 and postdoctoral work at the University of California, San Francisco in 2019.
Event Registration