Events
IFML Seminar
Reinforcement Learning for Highway Energy Efficiency: Deploying Cooperative Autonomous Vehicles at Scale
Eugene Vinitsky, Assistant Professor, NYU
-The Univeristy of Texas at Austin
Gates Dell Complex (GDC 6.302)
United States
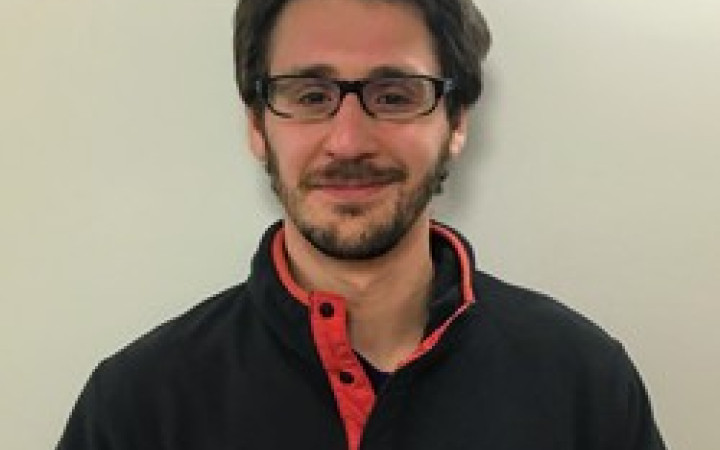
Abstract: The ever-increasing penetration of level-2 autonomous vehicles (AVs) offers an opportunity to reshape the energy efficiency and throughput of our highways. Even at current low penetration rates (1-5%), we have observed in small settings that adopting different driving behaviors from humans can sharply decrease fuel consumption by eliminating ubiquitous stop-and-go waves from traffic. We examined this idea at scale, showing that we can use reinforcement learning to design AV behaviors that operate cooperatively to smooth traffic in large, realistic simulators. We performed a large-scale benchmarking and showed that these controllers outperform analytical and model-based controllers. We then performed a large-scale road test, the first of its kind, in which we deployed a hundred of these cruise controllers onto a highway to show traffic smoothing at scale. While exact results for the fuel savings are ongoing, we have demonstrated changes in highway characteristics that are indicative of fuel improvements.
Speaker Bio: Eugene Vinitsky is an assistant professor in Transportation Engineering at NYU, a member of the C2SMARTER consortium on congestion reduction, and a part-time research scientist at Apple. He works primarily on multi-agent learning with a focus on its potential use in transportation systems and robotics. At UC Berkeley, where he was advised by Alexandre Bayen, he received his PhD in controls engineering with a specialization in reinforcement learning and received an MS and BS in physics from UC Santa Barbara and Caltech respectively. During his PhD he spent time at DeepMind, Tesla Autopilot, and FAIR.