IFML Researchers Take Home Two Outstanding Paper Awards at NeurIPS
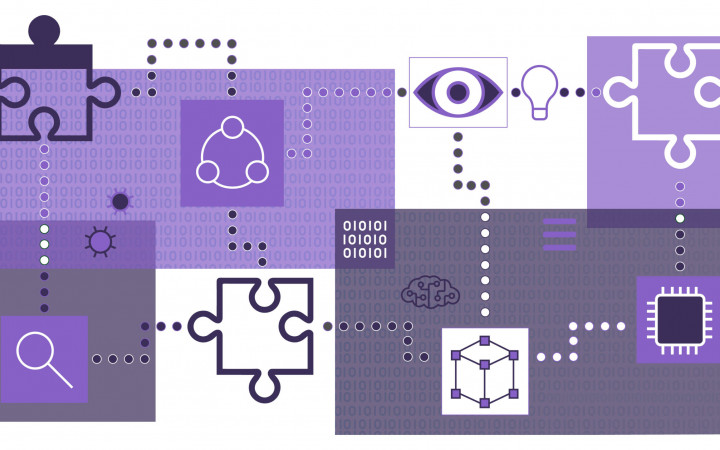
At the 2021 Conference on Neural Information Processing Systems, NeurIPS, six papers received Outstanding Paper Awards—two of them by IFML researchers. "A Universal Law of Robustness via Isoperimetry," co-authored by Sébastien Bubeck, and "MAUVE: Measuring the Gap Between Neural Text and Human Text using Divergence Frontiers," co-authored by Zaid Harchaoui.
Selected for "excellent clarity, insight, creativity, and potential for lasting impact," winning papers were announced via the NeurIPS blog along with summaries that detailed the award committee's selections.
A Universal Law of Robustness via Isoperimetry proposes a theoretical model to explain why many state-of-the-art deep networks require many more parameters than are necessary to smoothly fit the training data. In particular, under certain regularity conditions about the training distribution, the number of parameters needed for an O(1)-Lipschitz function to interpolate training data below the label noise scales as nd, where n is the number of training examples, and d is the dimensionality of the data. This result stands in stark contrast to conventional results stating that one needs n parameters for a function to interpolate the training data, and this extra factor of d appears necessary in order to smoothly interpolate. The theory is simple and elegant, and consistent with some empirical observations about the size of models that have robust generalization on MNIST classification. This work also offers a testable prediction about the model sizes needed to develop robust models for ImageNet classification.
MAUVE is a divergence measure to compare the distribution of model-generated text with the distribution of human-generated text. The idea is simple and elegant, and it basically uses a continuous family of (soft) KL divergence measures of quantized embeddings of the two texts being compared. The proposed MAUVE measure is essentially an integration over the continuous family of measures, and aims to capture both Type I error (generating unrealistic text) and Type II error (not capturing all possible human text). The empirical experiments demonstrate that MAUVE identifies the known patterns of model-generated text and correlates better with human judgements compared to previous divergence metrics. The paper is well-written, the research question is important in the context of rapid progress of open-ended text generation, and the results are clear.
Visit NeurIPS to learn more about this year's Outstanding Paper Awards.