IFML Researchers Win Two Outstanding Paper Awards at NeurIPS 2022
For the second consecutive year, IFML researchers won top honors at NeurIPS, the Conference and Workshop on Neural Information Processing Systems.
Jay Whang, a fourth-year Ph.D. student in Computer Science at UT Austin who is advised by IFML co-director Alex Dimakis, was part of the team that earned an outstanding paper award for Photorealistic Text-to-Image Diffusion Models with Deep Language Understanding. Conference judges stated: "This paper proposes a text-to-image diffusion model (Imagen) that generates high-fidelity images that accurately match the prompts. The work uses powerful language models (T5-XXL) that have been trained on text corpora that eventually aids in improved language understanding of the final model. Additionally, the paper makes modifications to the existing diffusion models by having dynamic thresholding along with some architectural changes to the U-Net to make it more efficient. With this work, Imagen becomes the state-of-the-art on MS-COCO based on the FID scores surpassing the models that are trained on it. The authors further introduce a benchmark, DrawBench, to evaluate the quality and accuracy of the image synthesis for a battery of challenging prompts. The results are impressive and of interest to a broad audience."
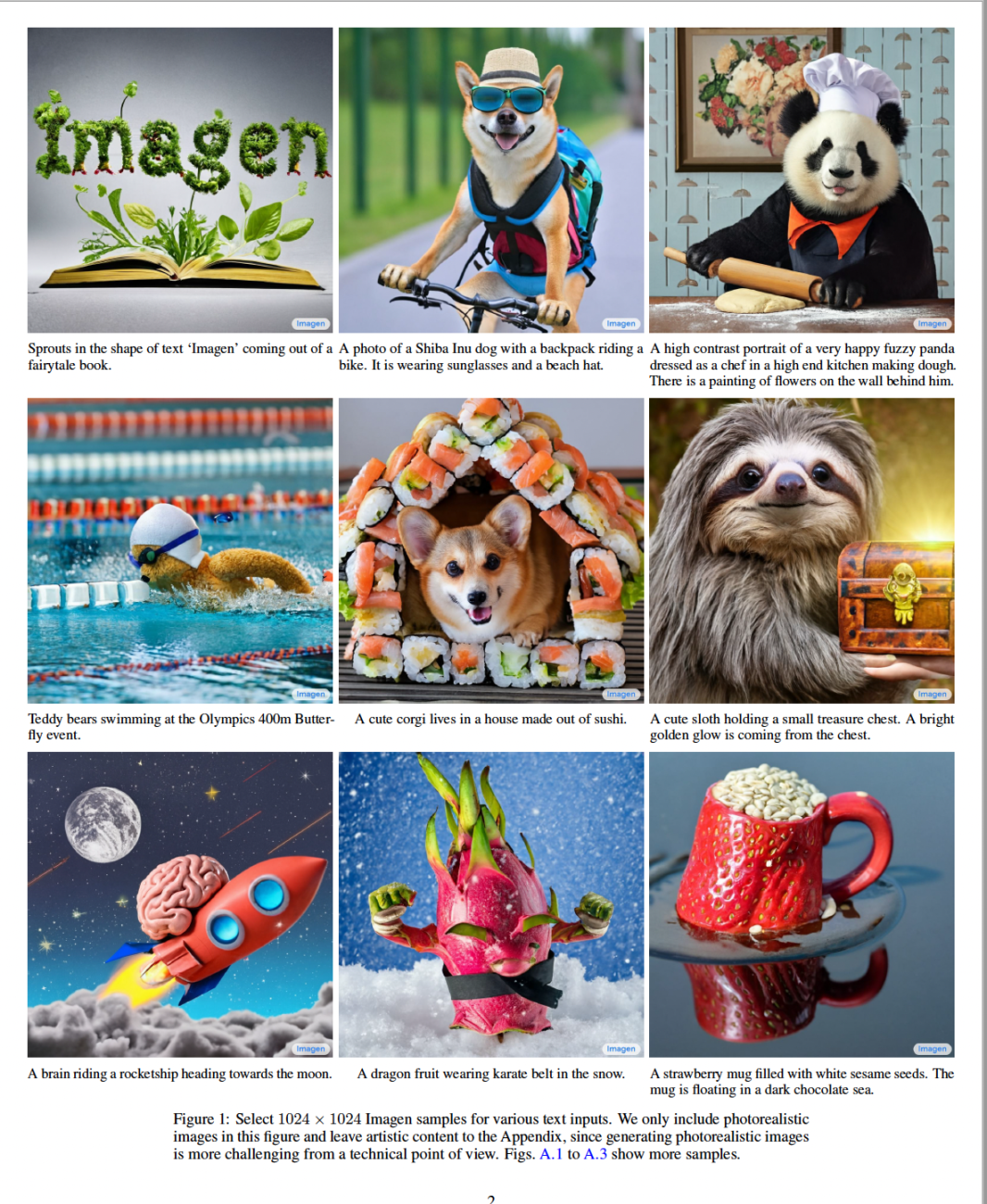
IFML member Ludwig Schmidt, assistant professor in computer science at the University of Washington, won In the Outstanding Datasets and Benchmarks Papers category for his contribution to LAION-5B: An open large-scale dataset for training next generation image-text models.
LAION-5B is an open, publicly available dataset of 5.8 billion CLIP-filtered image-text pairs, 14x bigger than LAION-400M, previously the largest openly accessible image-text dataset in the world. Studying the training and capabilities of language-vision architectures, such as CLIP and DALL-E, requires datasets containing billions of image-text pairs. Until now, no datasets of this size have been made openly available for the broader research community. This work presents LAION-5B, a dataset consisting of 5.85 billion CLIP-filtered image-text pairs, aimed at democratizing research on large-scale multi-modal models. NeurIPS judges noted, “the authors use this data to successfully replicate foundational models such as CLIP, GLIDE and Stable Diffusion, provide several nearest neighbor indices, as well as an improved web-interface, and detection scores for watermark, NSFW, and toxic content detection.”
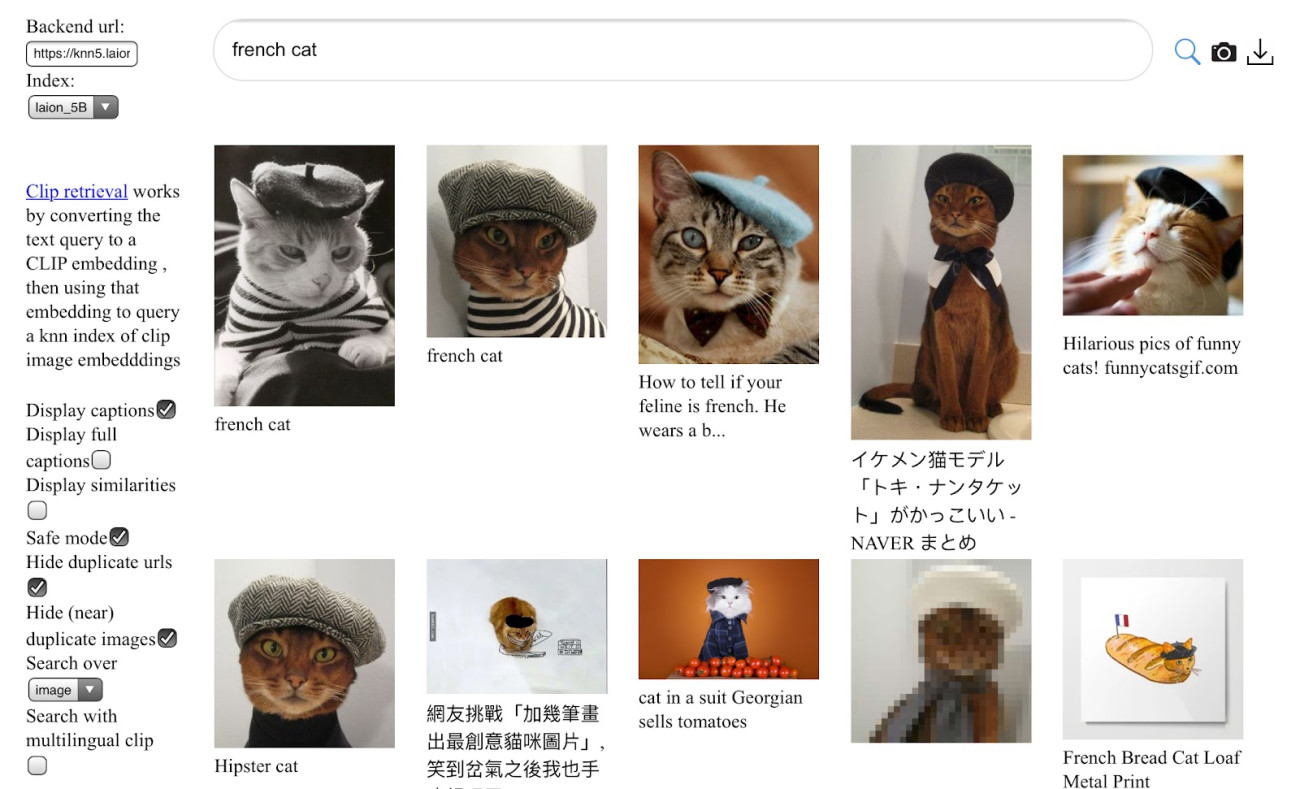
Many congratulations to the winners!