Knowledge-Augmented Contrastive Learning for Abnormality Classification and Localization in Chest X-rays with Radiomics using a Feedback Loop
Research thrust(s): Use-Inspired Applications
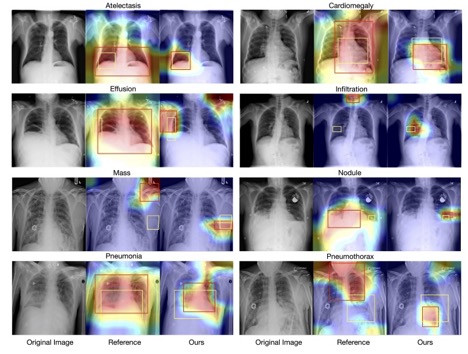
Chest X-rays are one of the most common radiological examinations for detecting cardiothoracic and pulmonary abnormalities. Due to the demand for accelerating chest X-ray analysis and interpretation, along with the overall shortage of radiologists, interest in building automated systems of chest X-ray abnormality classification and localization has been surging. While the class (i.e., outcomes) labels are important, the localization annotations, or the tightly bound local regions of images that are most indicative of the pathology, often provide richer information for clinical decision making (either automated or human-based). Automatic robust image analysis of chest X-rays currently faces many challenges. First, recognizing abnormalities in chest X-rays often requires expert radiologists. This process is therefore time-consuming and expensive to generate annotations for chest X-ray data, in particular the localized bounding box region labeling. Second, unlike natural images, chest X-rays have very subtle and similar image features. The most indicative features are hidden and localized. Therefore, chest X-rays are sensitive to distortion and not amendable to typical image data augmentations such as random cropping or color jittering. Moreover, in addition to high inter-class variance of abnormalities seen in chest X-rays (i.e., feature differences between different diseases), chest X-rays also have large intra-class variance (i.e., differences in presentation among individuals of the same diseases). The appearance of certain diseases in X-rays are often vague, can overlap with other diagnoses, and can mimic many other benign abnormalities. Last but not least, the class distribution of chest X-rays is also highly imbalanced for available datasets.
Fundamental algorithmic innovation: Contrastive Learning and Radiomics
Knowledge-Augmented Contrastive Learning is an innovative holistic framework that seamlessly integrates radiomic features as the other contrastive knowledge-augmentation for the chest X-ray image. As the main difference from existing frameworks, the two “views” that we contrast now are from two different domain knowledge characterizing the same patient: the chest X-ray image and the radiomic features. Notably, the radiomic features have to be extracted from the learned pathology localizations, which are not readily available. These features will be dynamically updated, forming a “feedback loop” during training in which both modalities’ learning mutually reinforce each other. The key enabling technique to link this feedback loop is a novel module we designed called Bootstrap Your Own Positive Samples (BYOP). For an unannotated X-ray image, we utilize Grad-CAM to generate the input heatmap from the image modality backbone, which yields the estimated bounding box after thresholding; and we then extract the radiomic features within this estimated bounding box, which becomes the alternative view to contrast with the image view. The usage of radiomic features also adds to the model interpretability.
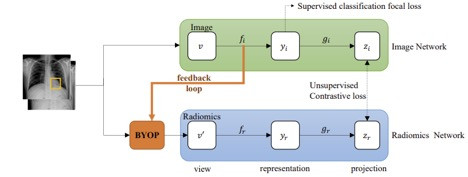
Our contributions are outlined as follows:
- A brand-new framework dedicated to improving abnormality identification and localization in (mostly unannotated) chest X-rays by knowledge-augmented contrastive learning, which highlights exploiting radiomic features as the auxiliary knowledge augmentation to contrast with the images, given the inability to perform classical image data augmentation.
- An innovative technique called BYOP to enable the effective generation of radiomic features, which is necessary as the true bounding boxes are often absent. BYOP leverages an interpretable learning technique to supply estimated bounding boxes dynamically during training.
- Excellent experimental results achieved on the NIH Chest X-ray benchmark, using very few annotations. Besides improving the disease classification AUC from 82.8% to 83.8%, our framework significantly boosts the localization results, by an average of 2% over different IoU thresholds, compared to reported baselines.
We propose a semi-supervised, end-to-end knowledge-augmented contrastive learning model that can jointly model disease classification and localization with limited localization annotation data. Our approach differs from previous studies in the choice of data augmentation, the use of radiomic features as prior knowledge, and a feedback loop for image and radiomic features to mutually reinforce each other. Additionally, the project aims to address current gaps in radiology by making prior knowledge more accessible to image data analytic and diagnostic assisting tools, with the hope that this will increase the model’s interpretability. Experimental results demonstrate that our method outperforms the state-of-the-art algorithms, especially for the disease localization task, where our method can generate more accurate bounding boxes. Importantly, we hope the method developed here is inspiring for the future research on incorporating different kinds of prior knowledge of medical images with contrastive learning.
Han, Y., Chen, C., Tewfik, A., Glicksberg, B., Ding, Y., Peng, Y., & Wang, Z. (2022). Knowledge-Augmented Contrastive Learning for Abnormality Classification and Localization in Chest X-rays with Radiomics using a Feedback Loop. Winter Conference on Applications of Computer Vision (WACV2022), Waikoloa, Hawaii, USA, Jan 4-8, 2022.
This research has been supported by the NSF AI Institute for Foundations of Machine Learning (IFML), Amazon Machine Learning Grant and the National Library of Medicine under Award No. 4R00LM013001.