Patch-VQ: 'Patching Up' the Video Quality Problem
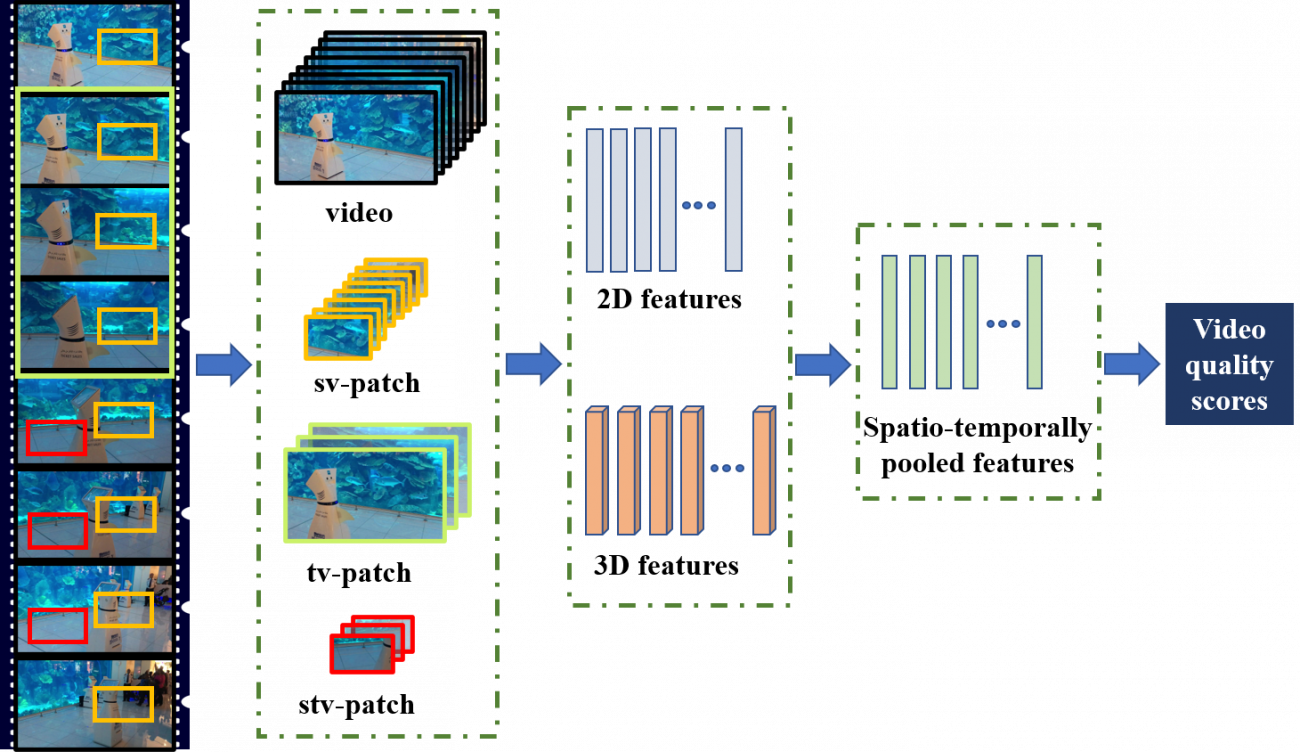
No-reference (NR) perceptual video quality assessment (VQA) is a complex, unsolved, and important problem to social and streaming media applications. Efficient and accurate video quality predictors are needed to monitor and guide the processing of billions of shared, often imperfect, user-generated content (UGC). Unfortunately, current NR models are limited in their prediction capabilities on real-world, "in-the-wild" UGC video data. To advance progress on this problem, we created the largest (by far) subjective video quality dataset, containing 38,811 real-world distorted videos and 116,433 space-time localized video patches ("v-patches"), and 5.5M human perceptual quality annotations. Using this, we created two unique NR-VQA models: (a) a local-to-global region-based NR VQA architecture (called PVQ) that learns to predict global video quality and achieves state-of-the-art performance on 3 UGC datasets, and (b) a first-of-a-kind space-time video quality mapping engine (called PVQ Mapper) that helps localize and visualize perceptual distortions in space and time.
LIVE-FB LSVQ Dataset
The LIVE-FB Large-scale Social Video Quality (LSVQ) Dataset includes 38,811 videos and 116,433 “v-patches” extracted from them, on which we collected about 5.5M quality scores in total from around 6,300 unique subjects. The dataset was created by sampling from 400,000 "UGC-like" videos from Internet Archive and Yahoo-Flicker Creative Commons 100M datasets.
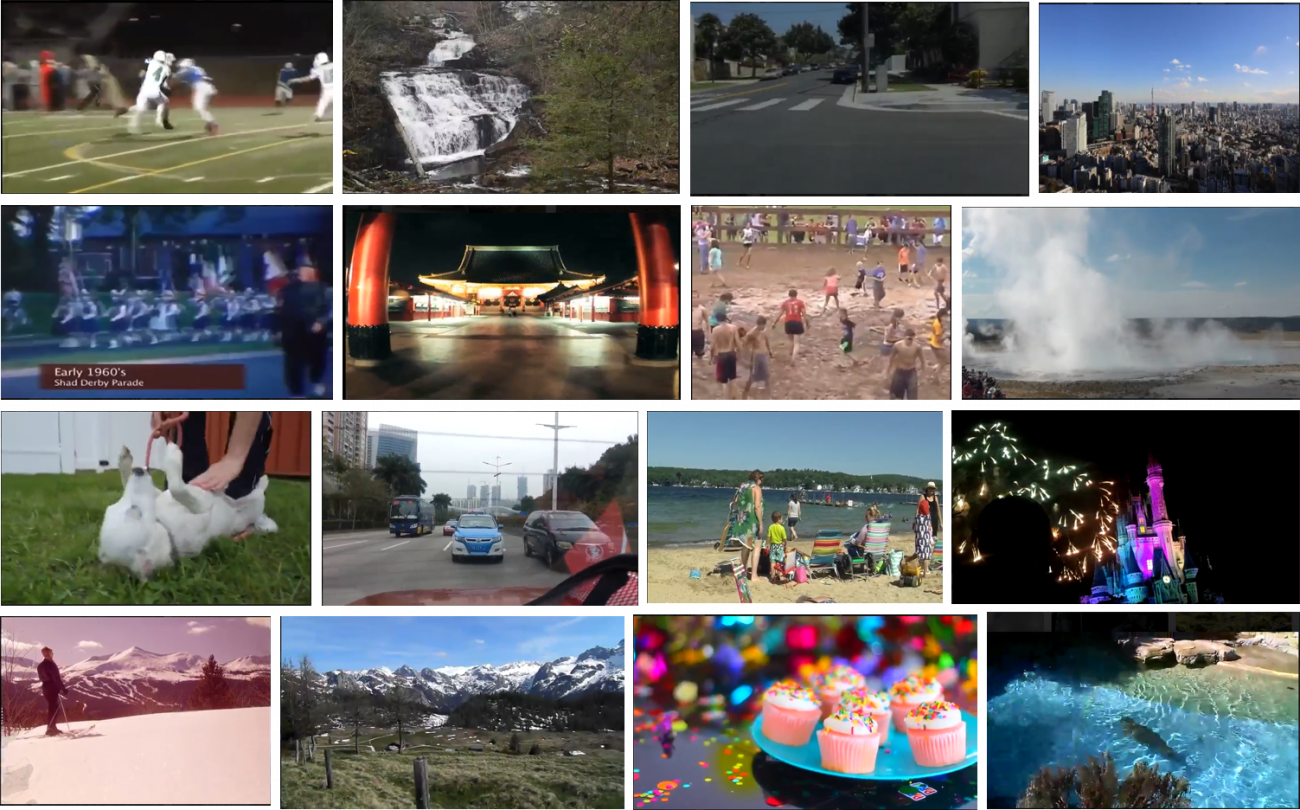
PVQ Mapper
We created a state-of-the-art deep blind video quality predictor, using a deep neural architecture that computes 2D and 3D video features. The features feed a time series regressor that learns to accurately predict both global video quality, as well as local space-time v-patch quality, by exploiting the relations between them. We also created another unique prediction model that predicts first-of-a-kind space-time maps of video quality. This second model, called the PVQ Mapper, helps localize, visualize, and act on video distortions.
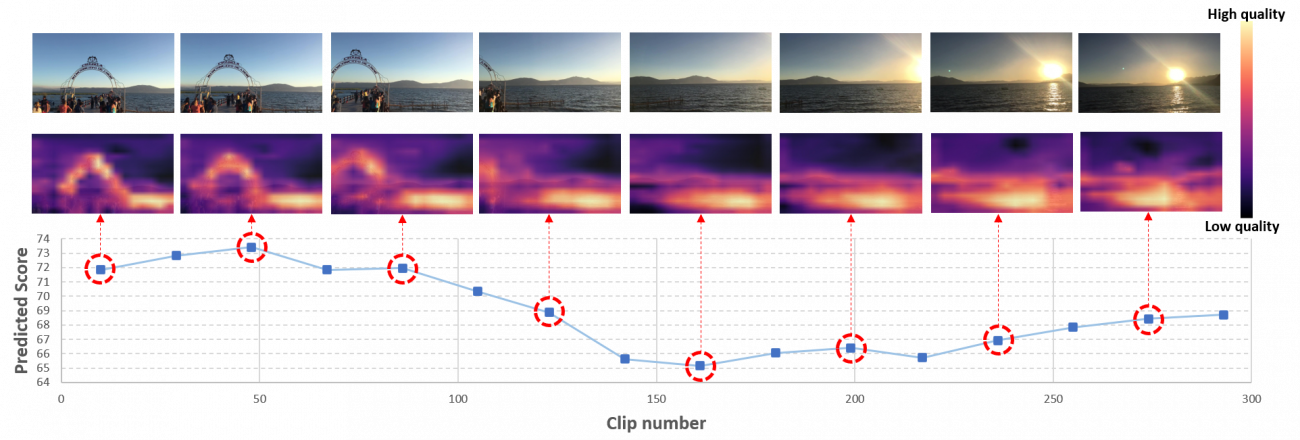
Examples
Generated Space-time Quality Maps (Video frames on the top, generated maps below)
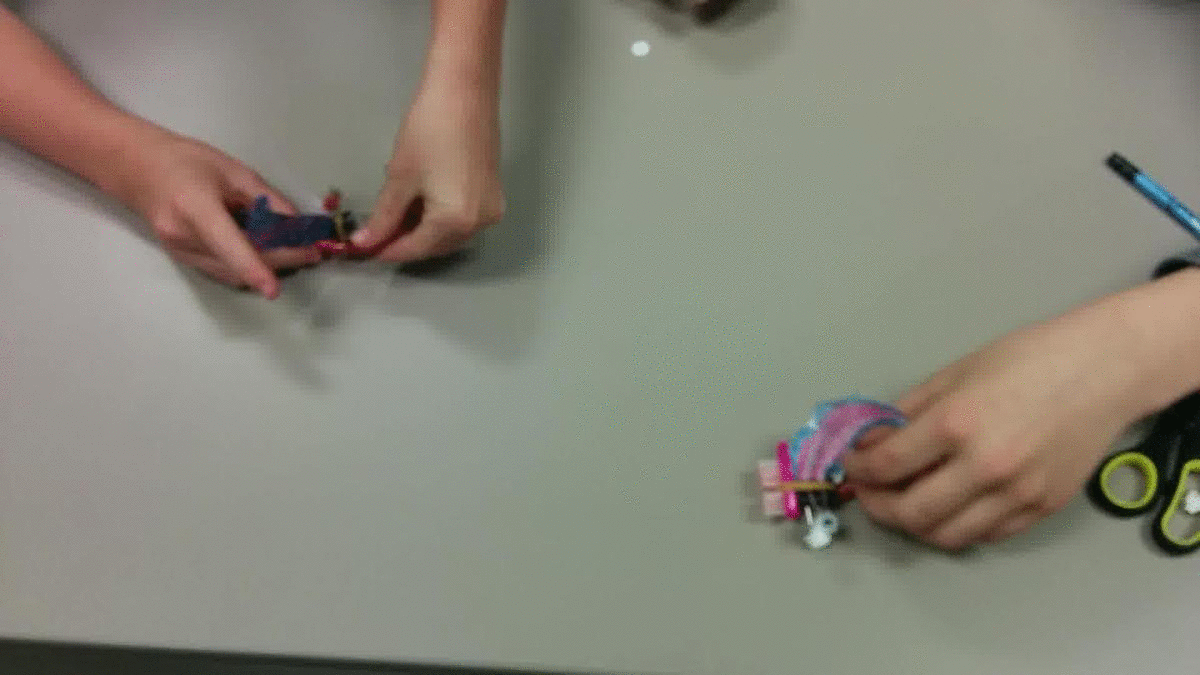
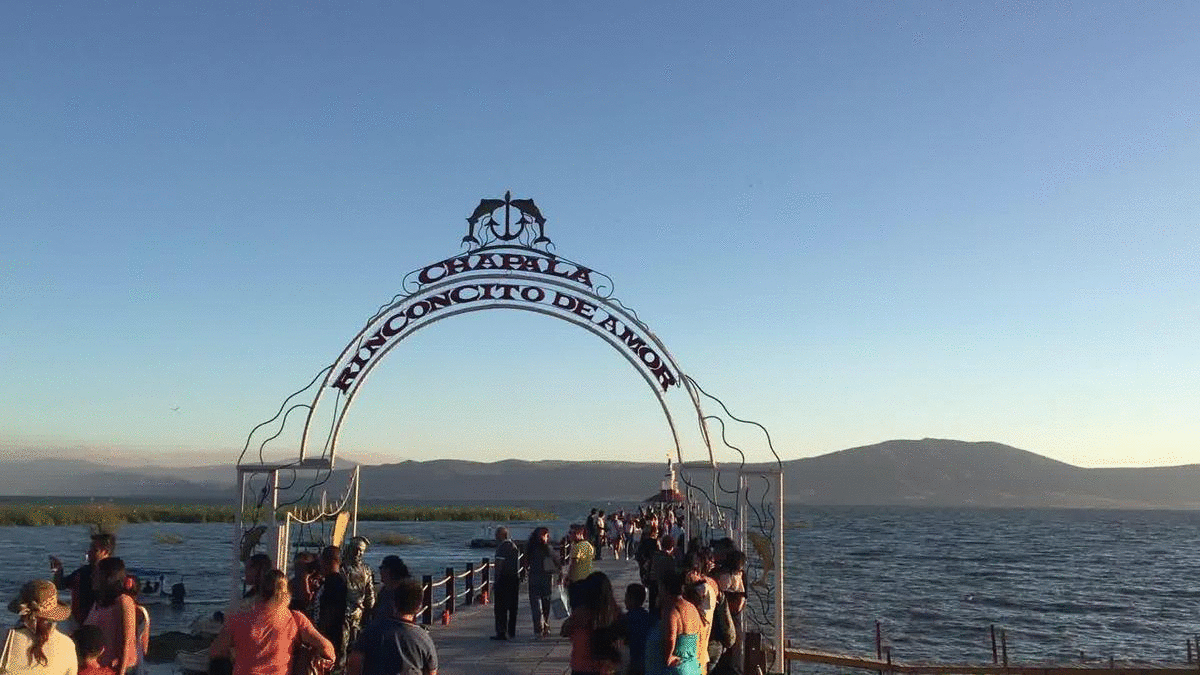
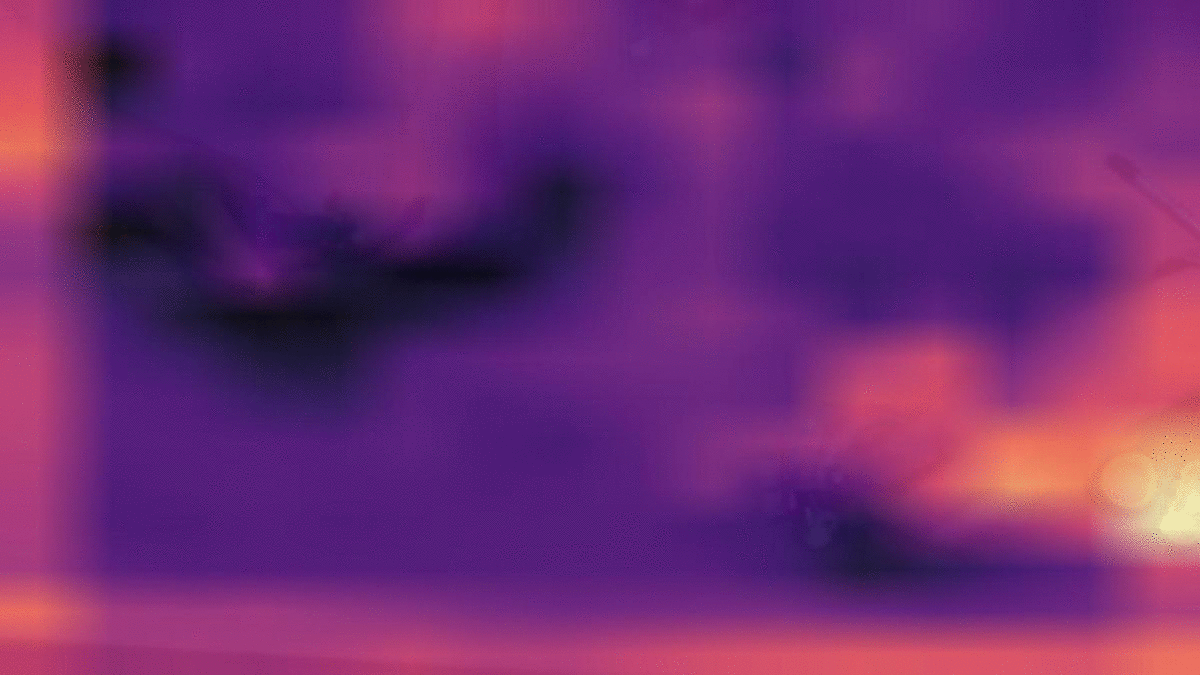
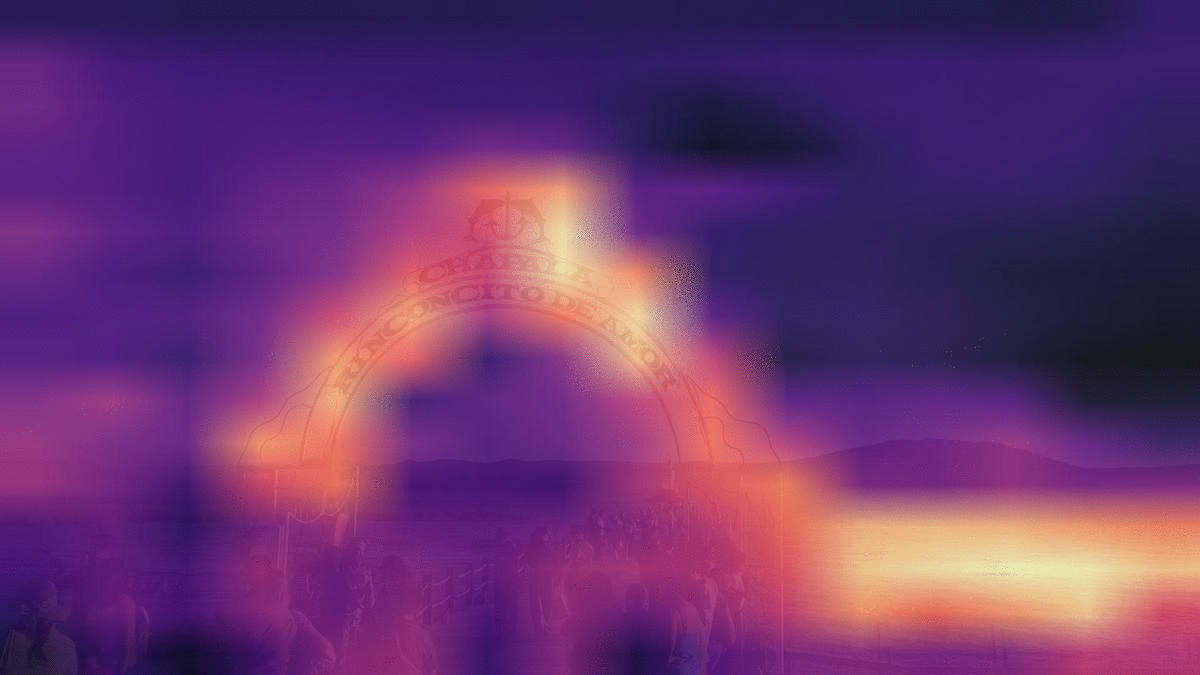
References
(1) Zhenqiang Ying, Maniratnam Mandal, Deepti Ghadiyaram, Alan Bovik Patch-VQ: 'Patching Up' the Video Quality Problem. In CVPR 2021 [Paper][Website]
(2) Zhenqiang Ying, Haoran Niu, Praful Gupta, Dhruv Mahajan, Deepti Ghadiyaram, Alan Bovik. From Patches to Pictures (PaQ-2-PiQ): Mapping the Perceptual Space of Picture Quality. In CVPR 2020 [Paper][Website]
(3) Vlad Hosu, Franz Hahn, Mohsen Jenadeleh, Hanhe Lin, Hui Men, Tamás Szirányi, Shujun Li, Dietmar Saupe. The Konstanz natural video database(KoNViD-1k). In Quality of Multimedia Experience (QoMEX) 2017 [Paper][Website]
(4) Zeina Sinno and Alan C. Bovik. Large-scale Study of Perceptual Video Quality. IEEE Transactions on Image Processing, vol. 28, no. 2, pp. 612-627, Feb. 2019 [Paper][Website]